Mgsv Upper Option Slot
Upper, side, and lower option slots Upgrading weapons keep saying that it gives me these, but unless I'm missing something, all the attachments like the laser sight and flashlight are automatically added to the weapon as its rank increases. My last good idea is to use my immense upper-body strength to hang precariously from the side of the base over the open ocean. My rival's feet clank on the walkways above. They’re sprinting.
The multi-armed bandit problem is a class example to demonstrate the exploration versus exploitation dilemma. This post introduces the bandit problem and how to solve it using different exploration strategies.
- What is Multi-Armed Bandit?
- Upper Confidence Bounds
The algorithms are implemented for Bernoulli bandit in lilianweng/multi-armed-bandit.
Exploitation vs Exploration
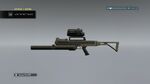
The exploration vs exploitation dilemma exists in many aspects of our life. Say, your favorite restaurant is right around the corner. If you go there every day, you would be confident of what you will get, but miss the chances of discovering an even better option. If you try new places all the time, very likely you are gonna have to eat unpleasant food from time to time. Similarly, online advisors try to balance between the known most attractive ads and the new ads that might be even more successful.
Fig. 1. A real-life example of the exploration vs exploitation dilemma: where to eat? (Image source: UC Berkeley AI course slide, lecture 11.)
If we have learned all the information about the environment, we are able to find the best strategy by even just simulating brute-force, let alone many other smart approaches. The dilemma comes from the incomplete information: we need to gather enough information to make best overall decisions while keeping the risk under control. With exploitation, we take advantage of the best option we know. With exploration, we take some risk to collect information about unknown options. The best long-term strategy may involve short-term sacrifices. For example, one exploration trial could be a total failure, but it warns us of not taking that action too often in the future.
What is Multi-Armed Bandit?
The multi-armed bandit problem is a classic problem that well demonstrates the exploration vs exploitation dilemma. Imagine you are in a casino facing multiple slot machines and each is configured with an unknown probability of how likely you can get a reward at one play. The question is: What is the best strategy to achieve highest long-term rewards?
In this post, we will only discuss the setting of having an infinite number of trials. The restriction on a finite number of trials introduces a new type of exploration problem. For instance, if the number of trials is smaller than the number of slot machines, we cannot even try every machine to estimate the reward probability (!) and hence we have to behave smartly w.r.t. a limited set of knowledge and resources (i.e. time).
Fig. 2. An illustration of how a Bernoulli multi-armed bandit works. The reward probabilities are unknown to the player.
A naive approach can be that you continue to playing with one machine for many many rounds so as to eventually estimate the “true” reward probability according to the law of large numbers. However, this is quite wasteful and surely does not guarantee the best long-term reward.
Definition
Now let’s give it a scientific definition.
A Bernoulli multi-armed bandit can be described as a tuple of (langle mathcal{A}, mathcal{R} rangle), where:
- We have (K) machines with reward probabilities, ({ theta_1, dots, theta_K }).
- At each time step t, we take an action a on one slot machine and receive a reward r.
- (mathcal{A}) is a set of actions, each referring to the interaction with one slot machine. The value of action a is the expected reward, (Q(a) = mathbb{E} [r vert a] = theta). If action (a_t) at the time step t is on the i-th machine, then (Q(a_t) = theta_i).
- (mathcal{R}) is a reward function. In the case of Bernoulli bandit, we observe a reward r in a stochastic fashion. At the time step t, (r_t = mathcal{R}(a_t)) may return reward 1 with a probability (Q(a_t)) or 0 otherwise.
It is a simplified version of Markov decision process, as there is no state (mathcal{S}).
The goal is to maximize the cumulative reward (sum_{t=1}^T r_t).If we know the optimal action with the best reward, then the goal is same as to minimize the potential regret or loss by not picking the optimal action.
The optimal reward probability (theta^{*}) of the optimal action (a^{*}) is:
[theta^{*}=Q(a^{*})=max_{a in mathcal{A}} Q(a) = max_{1 leq i leq K} theta_i]Our loss function is the total regret we might have by not selecting the optimal action up to the time step T:
[mathcal{L}_T = mathbb{E} Big[ sum_{t=1}^T big( theta^{*} - Q(a_t) big) Big]]Bandit Strategies
Based on how we do exploration, there several ways to solve the multi-armed bandit.
- No exploration: the most naive approach and a bad one.
- Exploration at random
- Exploration smartly with preference to uncertainty
ε-Greedy Algorithm
The ε-greedy algorithm takes the best action most of the time, but does random exploration occasionally. The action value is estimated according to the past experience by averaging the rewards associated with the target action a that we have observed so far (up to the current time step t):
[hat{Q}_t(a) = frac{1}{N_t(a)} sum_{tau=1}^t r_tau mathbb{1}[a_tau = a]]where (mathbb{1}) is a binary indicator function and (N_t(a)) is how many times the action a has been selected so far, (N_t(a) = sum_{tau=1}^t mathbb{1}[a_tau = a]).
According to the ε-greedy algorithm, with a small probability (epsilon) we take a random action, but otherwise (which should be the most of the time, probability 1-(epsilon)) we pick the best action that we have learnt so far: (hat{a}^{*}_t = argmax_{a in mathcal{A}} hat{Q}_t(a)).
Check my toy implementation here.
Upper Confidence Bounds
Random exploration gives us an opportunity to try out options that we have not known much about. However, due to the randomness, it is possible we end up exploring a bad action which we have confirmed in the past (bad luck!). To avoid such inefficient exploration, one approach is to decrease the parameter ε in time and the other is to be optimistic about options with high uncertainty and thus to prefer actions for which we haven’t had a confident value estimation yet. Or in other words, we favor exploration of actions with a strong potential to have a optimal value.
The Upper Confidence Bounds (UCB) algorithm measures this potential by an upper confidence bound of the reward value, (hat{U}_t(a)), so that the true value is below with bound (Q(a) leq hat{Q}_t(a) + hat{U}_t(a)) with high probability. The upper bound (hat{U}_t(a)) is a function of (N_t(a)); a larger number of trials (N_t(a)) should give us a smaller bound (hat{U}_t(a)).
In UCB algorithm, we always select the greediest action to maximize the upper confidence bound:
[a^{UCB}_t = argmax_{a in mathcal{A}} hat{Q}_t(a) + hat{U}_t(a)]Now, the question is how to estimate the upper confidence bound.
Hoeffding’s Inequality
If we do not want to assign any prior knowledge on how the distribution looks like, we can get help from “Hoeffding’s Inequality” — a theorem applicable to any bounded distribution.
Let (X_1, dots, X_t) be i.i.d. (independent and identically distributed) random variables and they are all bounded by the interval [0, 1]. The sample mean is (overline{X}_t = frac{1}{t}sum_{tau=1}^t X_tau). Then for u > 0, we have:
[mathbb{P} [ mathbb{E}[X] > overline{X}_t + u] leq e^{-2tu^2}]Given one target action a, let us consider:
- (r_t(a)) as the random variables,
- (Q(a)) as the true mean,
- (hat{Q}_t(a)) as the sample mean,
- And (u) as the upper confidence bound, (u = U_t(a))
Then we have,
[mathbb{P} [ Q(a) > hat{Q}_t(a) + U_t(a)] leq e^{-2t{U_t(a)}^2}]We want to pick a bound so that with high chances the true mean is blow the sample mean + the upper confidence bound. Thus (e^{-2t U_t(a)^2}) should be a small probability. Let’s say we are ok with a tiny threshold p:
[e^{-2t U_t(a)^2} = p text{ Thus, } U_t(a) = sqrt{frac{-log p}{2 N_t(a)}}]UCB1
One heuristic is to reduce the threshold p in time, as we want to make more confident bound estimation with more rewards observed. Set (p=t^{-4}) we get UCB1 algorithm:
[U_t(a) = sqrt{frac{2 log t}{N_t(a)}} text{ and }a^{UCB1}_t = argmax_{a in mathcal{A}} Q(a) + sqrt{frac{2 log t}{N_t(a)}}]Bayesian UCB
In UCB or UCB1 algorithm, we do not assume any prior on the reward distribution and therefore we have to rely on the Hoeffding’s Inequality for a very generalize estimation. If we are able to know the distribution upfront, we would be able to make better bound estimation.
For example, if we expect the mean reward of every slot machine to be Gaussian as in Fig 2, we can set the upper bound as 95% confidence interval by setting (hat{U}_t(a)) to be twice the standard deviation.
Fig. 3. When the expected reward has a Gaussian distribution. (sigma(a_i)) is the standard deviation and (csigma(a_i)) is the upper confidence bound. The constant (c) is a adjustable hyperparameter. (Image source: UCL RL course lecture 9’s slides)
Check my toy implementation of UCB1 and Bayesian UCB with Beta prior on θ.
Thompson Sampling
Thompson sampling has a simple idea but it works great for solving the multi-armed bandit problem.
Fig. 4. Oops, I guess not this Thompson? (Credit goes to Ben Taborsky; he has a full theorem of how Thompson invented while pondering over who to pass the ball. Yes I stole his joke.)
At each time step, we want to select action a according to the probability that a is optimal:
[begin{aligned}pi(a ; vert ; h_t) &= mathbb{P} [ Q(a) > Q(a'), forall a' neq a ; vert ; h_t] &= mathbb{E}_{mathcal{R} vert h_t} [ mathbb{1}(a = argmax_{a in mathcal{A}} Q(a)) ]end{aligned}]where (pi(a ; vert ; h_t)) is the probability of taking action a given the history (h_t).
For the Bernoulli bandit, it is natural to assume that (Q(a)) follows a Beta distribution, as (Q(a)) is essentially the success probability θ in Bernoulli distribution. The value of (text{Beta}(alpha, beta)) is within the interval [0, 1]; α and β correspond to the counts when we succeeded or failed to get a reward respectively.
First, let us initialize the Beta parameters α and β based on some prior knowledge or belief for every action. For example,
- α = 1 and β = 1; we expect the reward probability to be 50% but we are not very confident.
- α = 1000 and β = 9000; we strongly believe that the reward probability is 10%.
At each time t, we sample an expected reward, (tilde{Q}(a)), from the prior distribution (text{Beta}(alpha_i, beta_i)) for every action. The best action is selected among samples: (a^{TS}_t = argmax_{a in mathcal{A}} tilde{Q}(a)). After the true reward is observed, we can update the Beta distribution accordingly, which is essentially doing Bayesian inference to compute the posterior with the known prior and the likelihood of getting the sampled data.
[begin{aligned}alpha_i & leftarrow alpha_i + r_t mathbb{1}[a^{TS}_t = a_i] beta_i & leftarrow beta_i + (1-r_t) mathbb{1}[a^{TS}_t = a_i]end{aligned}]Thompson sampling implements the idea of probability matching. Because its reward estimations (tilde{Q}) are sampled from posterior distributions, each of these probabilities is equivalent to the probability that the corresponding action is optimal, conditioned on observed history.
However, for many practical and complex problems, it can be computationally intractable to estimate the posterior distributions with observed true rewards using Bayesian inference. Thompson sampling still can work out if we are able to approximate the posterior distributions using methods like Gibbs sampling, Laplace approximate, and the bootstraps. This tutorial presents a comprehensive review; strongly recommend it if you want to learn more about Thompson sampling.
Case Study
I implemented the above algorithms in lilianweng/multi-armed-bandit. A BernoulliBandit object can be constructed with a list of random or predefined reward probabilities. The bandit algorithms are implemented as subclasses of Solver, taking a Bandit object as the target problem. The cumulative regrets are tracked in time.
Fig. 4. The result of a small experiment on solving a Bernoulli bandit with K = 10 slot machines with reward probabilities, {0.0, 0.1, 0.2, …, 0.9}. Each solver runs 10000 steps. (Left) The plot of time step vs the cumulative regrets. (Middle) The plot of true reward probability vs estimated probability. (Right) The fraction of each action is picked during the 10000-step run.
Summary
We need exploration because information is valuable. In terms of the exploration strategies, we can do no exploration at all, focusing on the short-term returns. Or we occasionally explore at random. Or even further, we explore and we are picky about which options to explore — actions with higher uncertainty are favored because they can provide higher information gain.
Cited as:
Mgsv Upper Option Slot Machines
[1] CS229 Supplemental Lecture notes: Hoeffding’s inequality.
[2] RL Course by David Silver - Lecture 9: Exploration and Exploitation
[3] Olivier Chapelle and Lihong Li. “An empirical evaluation of thompson sampling.” NIPS. 2011.
[4] Russo, Daniel, et al. “A Tutorial on Thompson Sampling.” arXiv:1707.02038 (2017).
- 1Damage
- 2Drone upgrades
- 3EWAR
- 4Logistics
- 5Tackling
- 6Resource procurement
- 7Capital only
- 8Misc
High slots are a category of module slot found on ships in EVE. Generally, high slots contain the weapon systems of a ship, but there are plenty of other types of modules that use high slots, from mining lasers to drone upgrades.
This page is a collection of all the types of modules that use high slots.
Damage
These modules will deal direct damage to a target or multiple targets. The two basic weapon systems in the game are Turrets and Missile Launchers.
Hybrid turrets
- Main article: Turrets#Hybrid turrets
Hybrid turrets are the weapons used primarily by the Gallente. They use hybrid ammo that only deals thermal and kinetic damage.
Blasters | Railguns |
---|---|
Short range hybrid turrets. They have the most DPS of any type of weapon in game but also the least effective range. They are a bit easier to fit than railguns and are normally fitted on Gallente, and sometimes Caldari ships. | Long range hybrid turrets. They have longer range and higher rate of fire than other long-range weapon systems. They are moderately difficult to fit and are normally found on Gallente and some Caldari ships. |
Laser turrets
- Main article: Turrets#Laser turrets
Laser turrets are the weapons used primarily by the Amarr. They use frequency crystals as ammunition and deal EM and thermal damage. A special note to consider when fitting any ship is the ammunition usage. Lasers use crystals, which work differently than any other ammunition type. You can read more about it here.
Pulse laser turrets | Beam laser turrets |
---|---|
Short range laser turrets. They offer high damage potential while having decent range. They have fairly steep powergrid fitting requirements and are thus usually mounted only on Amarr ships. | Long range laser turrets. They have the highest damage potential and best tracking but the least effective range than other long-range weapon systems. They have the steepest fitting requirements of any weapon system, and will thus normally be fitted only on Amarr ships, although the smaller beam lasers are much easier to mount. |
Projectile turrets
- Main article: Turrets#Projectile turrets
Projectile turrets are the weapons used primarily by the Minmatar. They use no capacitor to fire and are very versatile.
Autocannons | Artillery |
---|---|
Short range projectile turrets. They have very good tracking and offer a flexible engagement range at the cost of DPS. Due to their versatility and low fitting requirements they will often be mounted on non-Minmatar ships. | Long range projectile turrets. They have the highest volley damage but the poorest tracking of all long-range weapon systems. They have the second-highest fitting requirements after beam lasers and are thus rarely found on non-Minmatar ships. |
Missile launchers
- Main article: Missile Launchers
Missile launchers are the primary weapon of the Caldari. Unlike turret-based weapon systems missile launchers have low powergrid needs but high CPU requirements. Many ships don't have enough turret hardpoints to fill a full rack of their preferred turret weapon, but will instead have launcher hardpoints. Like projectile turrets, missile launchers do not use capacitor to activate which makes them very versatile. However, launcher hardpoints are uncommon except on dedicated missile ships (certain Minmatar, Caldari, and a few T2 Amarr).
'Short-range' launchers | 'Long-range' launchers | Rapid Launchers |
---|---|---|
'Short-range' bays refers to missile launchers using the short-range, high damage missiles, namely rocket launchers, heavy assault missile launchers, torpedo launchers and citadel torpedo launchers. Except for rocket launchers, these short-ranged launchers have higher PG and CPU needs than their long-ranged counterparts (in contrast, long-ranged turrets have steeper fitting needs than their short-ranged counterparts). | 'Long-range' launchers refers to missile launchers using the long-range, low damage missiles, namely light missile launchers, heavy missile launchers, cruise launchers, and citadel cruise launchers. Except for Light Missile Launchers, these launchers have lower fitting needs than their close-ranged counterparts. | Rapid light and heavy missile launchers are different to their standard versions in several ways. Firstly, they're designed to be fitted to the ship class above, ie rapid light launchers are designed to be fitted to cruisers rather than frigates, and rapid heavy launchers are designed for battleships. Like the name suggests, they fire their missiles at a faster rate than the standard launchers, but apply their damage just as well. This makes rapid launchers a fantastic option for attacking smaller ships; a rapid light missile Caracal is one of the strongest anti-frigate ships around. It should also be noted that rapid missile launchers have a 40 second reload time; they can hit smaller ships for good damage, but take a long time to reload. |
Smartbombs
- Main article: Smartbombs
These weapons are area of effect weapons that hit everything within their activation range. They are particularly effective against drones and poorly equipped frigates. Smartbombs are available in sizes appropriate for frigates through battleships, but are seldom used on anything smaller than a battleship.
Bomb launchers
Mgsv Upper Option Slot Wins
- Main article: Bombs
Bomb launchers are used to launch bombs, which travel for a fixed distance and then explode for area of effect damage. They can only be fitted to Stealth Bombers.
Drone upgrades
There is only one module in the high slots which affects drones.
Drone Link Augmentors
These modules extend your drone control range. Drone Link Augmentors can be useful for drone ships and ships with nothing else to fit in their spare highslots.
EWAR
These modules will alter enemy ship capacitor. Energy destabilizers are great tools for removing an enemy ship's ability to repair itself, while energy vampires are useful for energy hungry ships and as a defense against hostile energy destabilization.
Energy Neutralizers
These modules will dissipate the capacitor of an enemy ship by using your capacitor energy.
Energy Vampires
These modules will transfer energy from the enemy ship to your capacitor, if your target's capacitor percentage level is higher than your own.
Logistics
These modules will restore cap, shields, armor, and hull to other ships.
These 4 module classes form the backbone of remote repair (RR) and are invaluable in PvP fleet battles, level 5 missions, incursions, and wormhole operations as single ships cannot be expected to withstand the assault of an enemy fleet.
Energy Transfer Arrays
These modules will transfer energy to the capacitor of an allied ship by using your capacitor energy.
Remote Armor Repair
These modules will repair the armor of an allied ship by using your capacitor energy.
Remote Hull Repair
These modules will repair the hull of an allied ship by using your capacitor energy.
Remote Shield Booster
These modules will repair the shield of an allied ship by using your capacitor energy.
Tackling
These modules will prevent ship warping.
Interdiction Sphere Launcher
These modules fire interdiction spheres which prevent warping and cannot be countered by warp core stabilizers. These modules are for interdictors.
Warp disruption Field Generator
These modules create a field around a heavy interdictor which prevent warping within a radius, but also slows the heavy interdictor and makes them incur more damage (via an increased signature radius).
Resource procurement
Several high slot modules are used to harvest material from objects in space, like asteroids, ice, and gas clouds
Mining Lasers
Mining lasers can be fitted on any ship with turret hardpoints and are used to mine ore. The regular mining lasers are capable of mining any type of ore except for Mercoxit which requires a deep core miner.
Strip Miners
Strip miners are bulk ore extractors that can only be fitted on mining barges and exhumers. They feature significantly longer cycle times but much more impressive extraction amounts, resulting in an improved yield over mining lasers in the majority of cases. The regular strip miners can mine any ore except for Mercoxit, while the deep core miner can mine all types of ore.
Ice Harvesters
Ice Harvesters harvest ice and can only be fitted on mining barges and exhumers.
Mgsv Upper Option Slot Machine
Gas Cloud Harvesters
Gas cloud harvesters harvest gas from gas clouds. They can be fitted on any ship with turret hardpoints.
Capital only
There are several modules that can only be fitted to capital or super capital ships. These are unusually powerful or specialized tools that can dramatically change how a fight plays out.Siege and triage modules are specialized modules mounted to certain capital ships that give them unique, very powerful properties, but at the cost of several drawbacks, the most important of which is that the capital ship is immobile for the duration of the siege module's cycle.
Siege Module
These are used by dreadnoughts to enter siege mode which massively increases their DPS, but makes them immobile, among other factors.
Triage Module
The triage module greatly increases a carrier's ability to provide assistance to a fleet while making it immobile, among other factors.
Industrial Core
The industrial core allows the Rorqual to compress ore.
Drone Control Units
These modules allow the ship to control one extra drone each. Can only be fitted by carriers and supercarriers.
Remote ECM Burst
These modules will emit an area of effect ECM burst, centered on a target which has a chance to break the lock of all ships within its range. These can only be fitted onto supercarriers.
Clone Vat Bay
Clone Vat Bay can only be fitted on titans and the Rorqual. It allows for the installation of jump clones, enabling clone-jumping to the ship with the bay.
Jump Portal Generators
The regular jump portal generator can only be fitted on a titan and is used to create jump bridges allowing your fleetmates to quickly traverse vast distances without the need for stargates.
Doomsday devices
Doomsday devices are extremely powerful weapons mounted on titans. They deal 2 million points of racial type damage to a single target while using up 50 thousand units of the racial isotope to do so. They also have several drawbacks such preventing the titan from using a warp or jump drive for ten minutes after using the doomsday.
Misc
These modules did not fit into the above categories.
Auto Targeting
These modules will target for you, and increase your maximum targeted ships by one. Generally, these are dangerous to activate, as it is important to be aware of who you have targeted, especially in high sec.
Bastion Module
A siege type module for marauders. Using it immobilizes the ship and prevents remote assistance for 60 seconds, but grants increased weapon range, immunity to electronic warfare, and a big bonus to tank.
Covert Jump Portal Generator
Used by Black Ops battleships to create a covert jump bridge, which allows fleetmates to travel to other systems instantaneously. Only ships that can equip covert ops cloaking devices can use this jump bridge.
Cynosural Field Generators
These modules will create a cynosural field that will allow capital ships to enter a system. Be careful, as using the cyno field generator will leave your ship immobile for ten (10) minutes. Cynosural field generators can be used only on Force Recon cruisers and black ops battleships. The covert cynosural field generator works in a similar manner, except it can only be used by black ops ship. Industrial cynosural field generator is another variant that is only usable on hauling ships.
Cyno field generators work best on an alt in a ship that you don't mind losing, because as soon as the cyno field is created, everyone in the system knows where you are.
Cloaking
These modules will make you invisible unless you use a module or warp, or come within 2km of something. The Covert Ops Cloak for recon ships and covert ops ships will not decloak for warp. These are invaluable for scouts.
Mgsv Upper Option Slot No Deposit
Entosis Link
Entosis Links are used to capture sovereignty in null sec.
Fleet assistance modules
- Main article: Command Bursts
These activatable modules will give bonuses to fleet members within range. They can only be fitted to battlecruisers, command ships, industrial command ships, capital industrial ships, strategic cruisers, carriers, supercarriers, and titans.
Salvager
These modules will salvage loot from wrecks.
Scan Probe Launchers
These modules will launch scan probes to allow you to explore.
Tractor Beams
These modules will bring cargo containers and wrecks to your ship.